By Bob O’Donnell
In case you hadn’t noticed, AI is having a year.
In fact, the explosion of interest and attention directed at generative AI applications like ChatGPT as well as major new initiatives from the likes of Microsoft, Google, Amazon, IBM, Salesforce, and more is driving enterprise IT departments into scramble mode. It’s no longer a question of whether to integrate more AI-based capabilities into their organization, it’s now just an issue of how and when.
But more than that, how does an organization mobilize its data, and how should it develop true differentiation? Every organization has mountains of under-used data, with latent potential. What data do they have? Structured data, including customer transactions and operational metrics. Unstructured data, such as contracts, documents, images and more. What insights could enhance their core business, and what information could be shared with partners? We’ve learned how we can harness that untapped data, and use AI/ML to drive smarter decision making and accelerate innovation.
However, as has been discussed throughout this blog series, there are quite a few issues that need to be addressed to do this in a productive, useful and safe manner. All the various components of the hardware infrastructure necessary to power AI-enabled applications or AI models need to be optimized for the kinds of specific demands that AI-based workloads require.
Companies also need to think through the key principles that will drive their data strategy in relation to AI. For one, it’s clear that AI models and applications of all types need access to very large amounts of data. While some companies may have initially thought that a cloud-based approach would give them more flexibility, many are starting to realize the costs involved with moving large amounts of data to and from the cloud are prohibitive. As a result, there’s a strong move towards companies keeping critical data sets that can be used for latency-sensitive AI applications on premises to avoid data migration charges. This entails the need to have powerful storage systems within the company’s datacenter or at co-location site. At the same time, data demands for an organization can also go up and down depending on the projects at hand. As a result, many companies are attracted by the agility offered by elastic or cloud storage.
From Enterprise Data Lakehouse to AI Workhorse
When it comes to storage architectures, many organizations have started to recognize the importance and value of a data lakehouse as an operational store for regular enterprise applications. In essence, a data lakehouse combines the capabilities of traditional data warehouses and data lakes. Like data lakes, a data lakehouse can store structured data like spreadsheets and transaction data that data warehouses are limited to, along with the growing amount of semi-structured and unstructured data (documents, email, audio, video, etc.) that many companies now also retain. On top of that, a data lakehouse adds the ability to do things like traditional data warehouse-style queries on unstructured or semi-structured data, which makes them especially useful for data transformation, data labelling and data preparation stages in an AI pipeline.
With AI-based workloads—whether it’s more traditional machine learning-based data analytics or new training and inferencing workloads associated with building or refining foundation models—the flexibility to decide how and where to deploy stages of the AI data delivery pipeline becomes even more apparent.
The increased variety and amount of operational data that can be drawn from an enterprise lakehouse makes it a powerful source for AI training data. This is particularly true for companies who are either building their own foundation models or doing additional training on existing models.
Even with traditional machine-learning style data analytics used in non-generative AI applications, this flexibility and scalability can make an important difference in the effectiveness of the algorithms that are generated from this data.
From Smarter decision-making to Generative AI
Thanks to the impressive strides that generative AI is making in general productivity as well as industry-specific solutions, the focus on AI applications in business is unquestionably going to grow. One of the more exciting new areas is the ability for organizations to customize existing foundation models and use them as private, internal tools. Many companies find this concept appealing because they can leverage the enormous amount of effort that’s gone into creating these models but avoid the potential data leakage/intellectual property (IP) loss issues that have already hit the headlines.
Some companies are also starting to look at leveraging the enormous range of open-source AI models and data sets from companies like Hugging Face. These provide yet another alternative for companies who want to tap into the power of AI applications without reinventing the wheel on general-purpose capabilities like large language models (LLMs).
Regardless of the approach that companies choose to pursue, it seems obvious that over the next 12-24 months there’s going to be an enormous number of new AI-driven workloads being run at companies around the world. The new transformer model-driven generative AI tools have already had a striking impact on the tech industry and companies in other industries around the world are starting to appreciate their potential impact as well.
Adopting a Data-First AI Strategy
For organizations considering how best to harness their untapped data, the most important step is to uncover the data sources which could be mobilized, both structured and unstructured. An existing data lakehouse is an ideal source repository, to which can be added data labelling to construct training and validation datasets, ready to transfer to the high-performance shared platforms for their data engineers to build, train and test AI models.
Moving forward, we will increasingly see elastic, distributed storage systems which will bridge the gap from today’s data lakehouse technologies to deliver the performance needed for AI learning at the scale needed for high-throughput deep learning.
In order to be best prepared for these efforts, companies obviously have to think through the computing requirements they will need, but equally important are the data storage tools and strategies that will be required for AI data management. Exactly where this will all lead isn’t completely clear, and there are definitely still some important obstacles to overcome, but the future of enterprise computing hasn’t looked this exciting for quite some time.
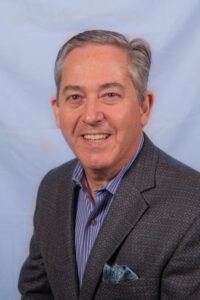
Bob O’Donnell is the president and chief analyst of TECHnalysis Research, LLC a market research firm that provides strategic consulting and market research services to the technology industry and professional financial community. You can follow him on Twitter @bobodtech.